
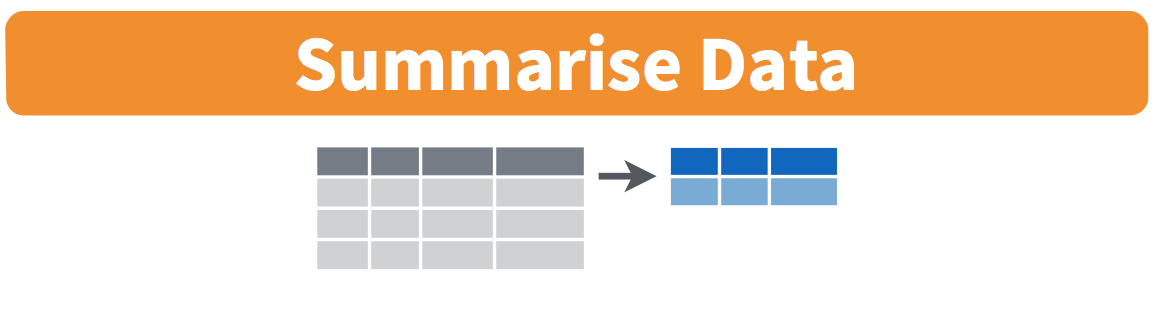
1/3 of them are female, and 2/3 are male. It is a group of middle-age wealthy people. There is a lot of information you can extract from those simple averages.Ĭonspicuous: average age is about 40.
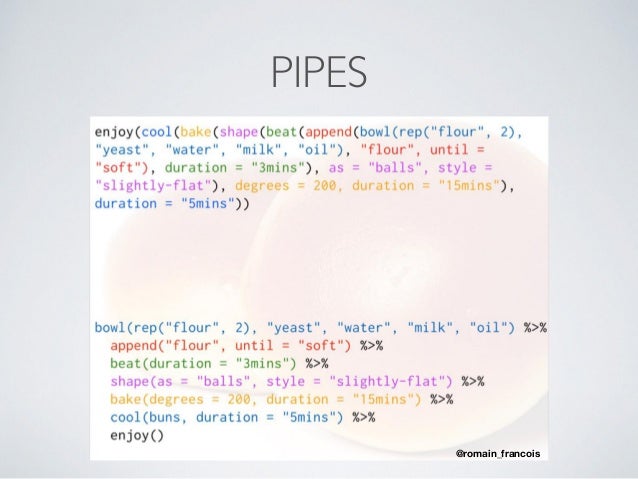
The third argument summarise tells R the manipulation(s) to do. Here we only summarize data by one categorical variable, but you can group by multiple variables, such as group_by(segment, house). The second line group_by(segment) tells R that in the following steps you want to summarise by variable segment.
#DPLYR SUMMARIZE MEAN BY GROUP CODE#
Now, let’s look at the code in more details. 14.1 Customer Data for Clothing Companyĭat_summary % dplyr :: group_by(segment) %>% dplyr :: summarise( Age = round( mean( na.omit(age)), 0), FemalePct = round( mean(gender = "Female"), 2), HouseYes = round( mean(house = "Yes"), 2), store_exp = round( mean( na.omit(store_exp), trim = 0.1), 0), online_exp = round( mean(online_exp), 0), store_trans = round( mean(store_trans), 1), online_trans = round( mean(online_trans), 1)) # transpose the data frame for showing purpose # due to the limit of output width cnames % ame() names(tdat_summary) 12.1.1 Logistic Regression as Neural Network.11.4 Regression and Decision Tree Basic.10.4 Penalized Generalized Linear Model.9.2 Principal Component Regression and Partial Least Square.9.1.2 Diagnostics for Linear Regression.6.1.2 apply(), lapply() and sapply() in base R.5.2.1 Impute missing values with median/mode.4.3.1 Open Account and Create a Cluster.3.1 Customer Data for a Clothing Company.2.5.4 Model Implementation and Post Production Stage.2.4.4 Model Implementation and Post Production Stage.
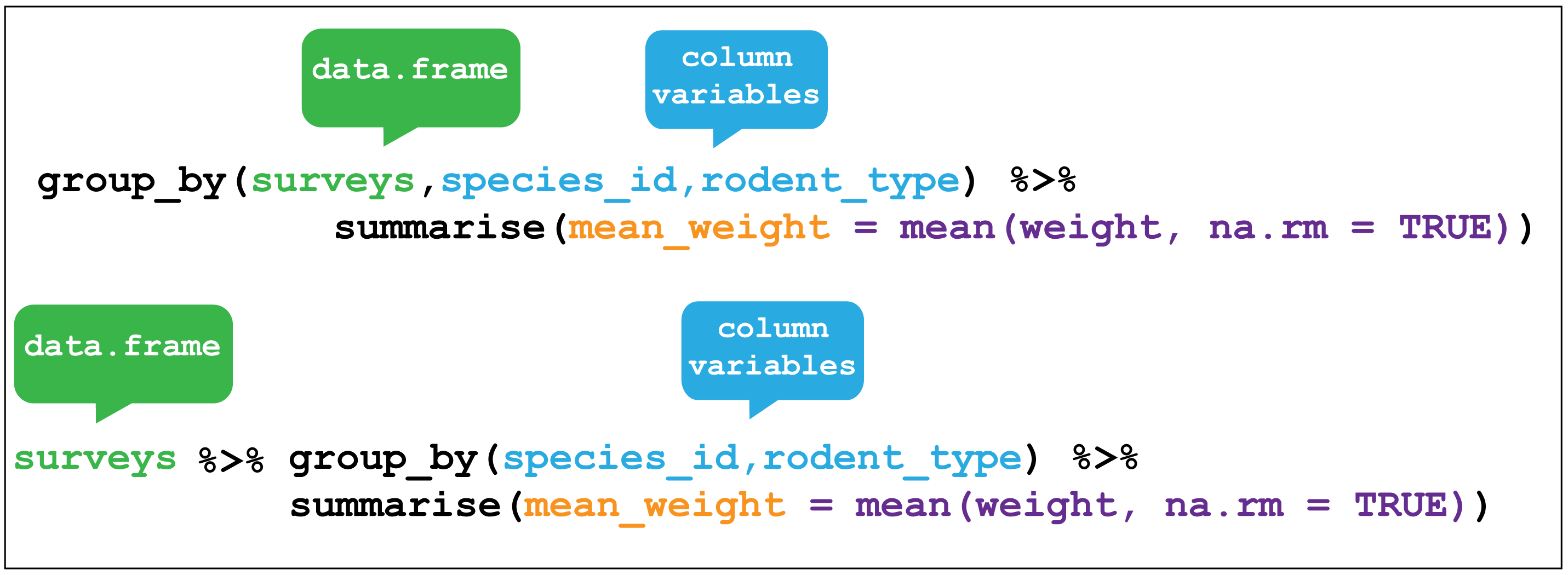
2.4.2 Problem Formulation and Project Planning Stage.2.1 Comparison between Statistician and Data Scientist.1.3 What Kind of Questions Can Data Science Solve?.
